Statistically Sound Machine Learning for Automated Trading of Financial Instruments: A Comprehensive Guide

The advent of machine learning (ML) has revolutionized various industries, including the financial sector. Automated trading, powered by ML algorithms, has become increasingly prevalent as it offers the potential for better decision-making, reduced trading costs, and enhanced risk management. However, to effectively utilize ML for automated trading, it is crucial to adhere to statistically sound principles and best practices. This comprehensive guide will delve into the essential aspects of statistically sound machine learning for automated trading of financial instruments.
The foundation of successful ML models lies in high-quality data. For automated trading, this entails collecting and cleaning vast amounts of financial data, including historical prices, economic indicators, and market news. The data should be preprocessed to address outliers, missing values, and data inconsistencies. Feature engineering techniques can be employed to extract relevant features and create informative input variables for the ML models.
The choice of ML model depends on the specific trading strategy and the characteristics of the financial data. Supervised learning algorithms, such as linear regression, support vector machines, and decision trees, are commonly used for predicting price movements. Ensemble methods, such as random forests and gradient boosting, can improve model performance by combining multiple weak learners.
4.1 out of 5
Language | : | English |
File size | : | 27822 KB |
Model evaluation is essential to assess the performance and reliability of the ML models. Cross-validation techniques are used to estimate the generalization error and prevent overfitting. Evaluation metrics specific to financial trading, such as Sharpe ratio, profit factor, and maximum drawdown, should be considered.
Statistical inference plays a vital role in validating the significance and robustness of trading models. Hypothesis testing can be employed to determine whether there is a statistically significant relationship between the model's predictions and the observed outcomes. Statistical significance tests, such as the t-test and ANOVA, can be used to assess the statistical validity of model performance metrics.
Risk management is paramount in automated trading. ML models should be integrated with robust risk management strategies to mitigate potential losses. This involves setting stop-loss orders, calculating position sizing, and managing risk exposure. Optimization techniques, such as mean-variance optimization and portfolio optimization, can be used to allocate capital efficiently and enhance overall portfolio performance.
Backtesting involves evaluating the performance of the ML model on historical data to gauge its potential effectiveness. Simulated trading environments can be created to test the model's robustness under different market conditions. Backtesting and simulation provide valuable insights into the model's strengths, weaknesses, and areas for improvement.
Once the ML model has been thoroughly tested and validated, it can be deployed for live trading. Continuous monitoring is crucial to track model performance, identify potential issues, and make necessary adjustments. Automated trading systems should be equipped with robust monitoring mechanisms to detect any anomalies or performance deterioration.
Automated trading using ML algorithms raises ethical considerations regarding market manipulation and the potential for unfair advantage. It is imperative to adhere to ethical guidelines and best practices to ensure transparency, fairness, and market integrity.
Statistically sound machine learning is a powerful tool for automated trading of financial instruments. By adhering to the principles outlined in this guide, traders can develop and deploy robust trading models that effectively leverage market data and make informed trading decisions. Continuous research and innovation in ML algorithms and statistical methods will further enhance the capabilities of automated trading systems, paving the way for even greater success in the financial markets.
Relevant Long Descriptive Keywords for Alt Attribute:
- Statistical Machine Learning for Automated Trading
- Data Quality and Preparation for Financial Trading
- Model Selection and Evaluation for Trading Models
- Statistical Inference and Hypothesis Testing in Financial ML
- Risk Management and Optimization for Automated Trading
- Backtesting and Simulation for Trading Strategies
- Ethical Considerations in Automated Trading
Long Tail Title:
A Comprehensive Guide to Statistically Sound Machine Learning for Automated Trading of Financial Instruments: Maximizing Efficiency, Mitigating Risk, and Achieving Success
4.1 out of 5
Language | : | English |
File size | : | 27822 KB |
Do you want to contribute by writing guest posts on this blog?
Please contact us and send us a resume of previous articles that you have written.
Book
Novel
Page
Chapter
Story
Reader
Library
Magazine
Shelf
Preface
Synopsis
Manuscript
Scroll
Codex
Bestseller
Narrative
Autobiography
Memoir
Reference
Encyclopedia
Dictionary
Thesaurus
Narrator
Catalog
Card Catalog
Borrowing
Periodicals
Study
Research
Reserve
Academic
Journals
Reading Room
Literacy
Study Group
Thesis
Storytelling
Reading List
Theory
Textbooks
Tom Tidman
Sotiris Bakanakis
Edina Balczo
Elisa Downing
Susie Kelly
D Marvin Jones
Sidney G Tarrow
Frankie Poullain
Jim Knight
Eric R Dodge
Andy Belcher
Tim Lavers
Audrey Niffenegger
Jan Pienkowski
John Atkinson Abutridy
Bernard Sanders
David F Burg
Mimi Thi Nguyen
Zachary Hubbard
Karen Harper
Light bulbAdvertise smarter! Our strategic ad space ensures maximum exposure. Reserve your spot today!
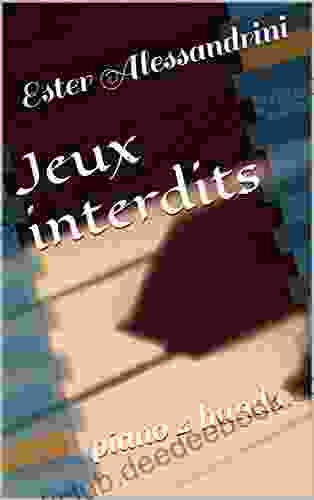

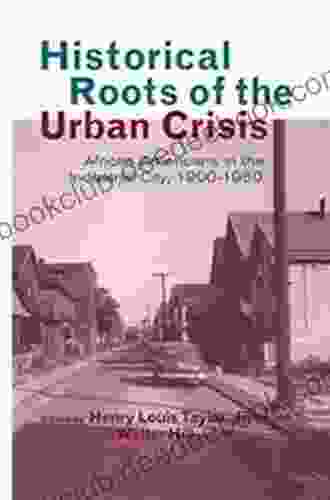

- Benjamin StoneFollow ·17.8k
- Billy FosterFollow ·15.8k
- Harrison BlairFollow ·16.2k
- Ian MitchellFollow ·15k
- Lawrence BellFollow ·19.4k
- Dillon HayesFollow ·9.6k
- Robert Louis StevensonFollow ·8.4k
- Chase MorrisFollow ·11.2k
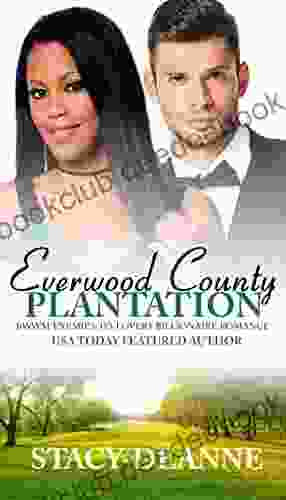

BWWM Enemies to Lovers Billionaire Romance: A Captivating...
In the realm of romance novels, the...
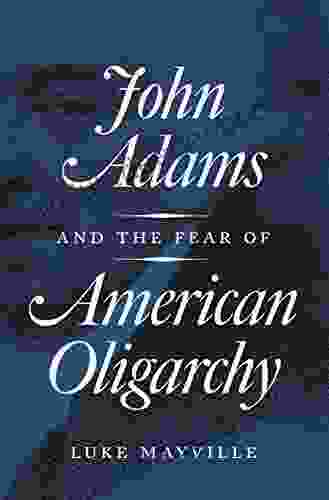

John Adams and the Fear of American Oligarchy
John Adams, a...
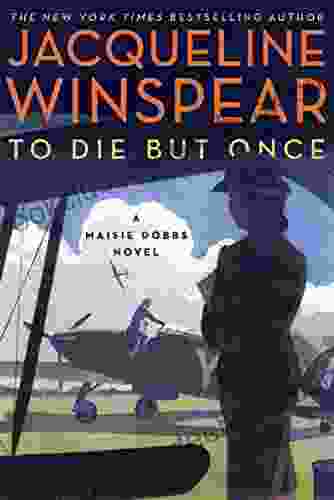

To Die but Once: A Haunting Maisie Dobbs Novel
Synopsis ...
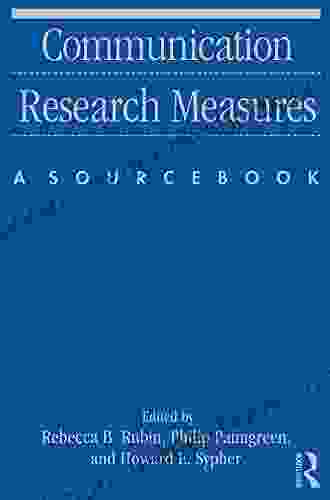

Communication Research Measures Sourcebook Routledge...
Communication research measures are the...
4.1 out of 5
Language | : | English |
File size | : | 27822 KB |